Lessons from space communication and AI tackle the reproducibility crisis
VIB Bioimaging experts address the difficulties of reproducibility by turning metadata redundancy into an error correction tool, with a little help from AI.
The reproducibility crisis
One of the core principles of the scientific method is the validation of results. To achieve this, scientists would ideally run their tests or experiments again to see whether the results the first time weren't a coincidence. Even better would be if other scientists could independently validate the results. That way, we can rule out that there are specific (lab) conditions that skew the results. The independent replication of scientific results is the strongest clue we have that a finding reveals something true about reality.
But, for a result to be replicated, the research behind it needs to be reproducible. To make sure that research results can be reproduced by others, scientists share their methods and protocols. That, however, is easier said than done. A large 2016 survey by the journal Nature revealed that over 70% of researchers struggle to reproduce experiments. This reproducibility crisis can be attributed to factors like limited access to raw data, insufficient documentation, and – in this age of big data – the complexities of managing vast datasets.
Now, in an effort spearheaded by Tatiana Woller, Data Expert at the VIB Bioimaging Core Leuven, VIB researchers look at unlikely inspirations to mitigate the reproducibility crisis: space communication and AI.
"In bioimaging," says Sebastian Munck, Innovation Technologist at the VIB Bioimaging Core Leuven, "several initiatives and standards, notably the FAIR principles (Findable, Accessible, Interoperable, Reusable), aim to improve the quality of the data, and as a result, the reproducibility of the research. But the need for data annotations to adhere to these standards can lead to so-called metadata redundancy."
"So," Tatiana Woller continues, "why not put that metadata to good use? In space communication, metadata redundancy is used for error correction. What if we can turn that redundancy in bioimaging data documentation into a tool for consolidating information and enhancing the reproducibility of bioimaging experiments?"
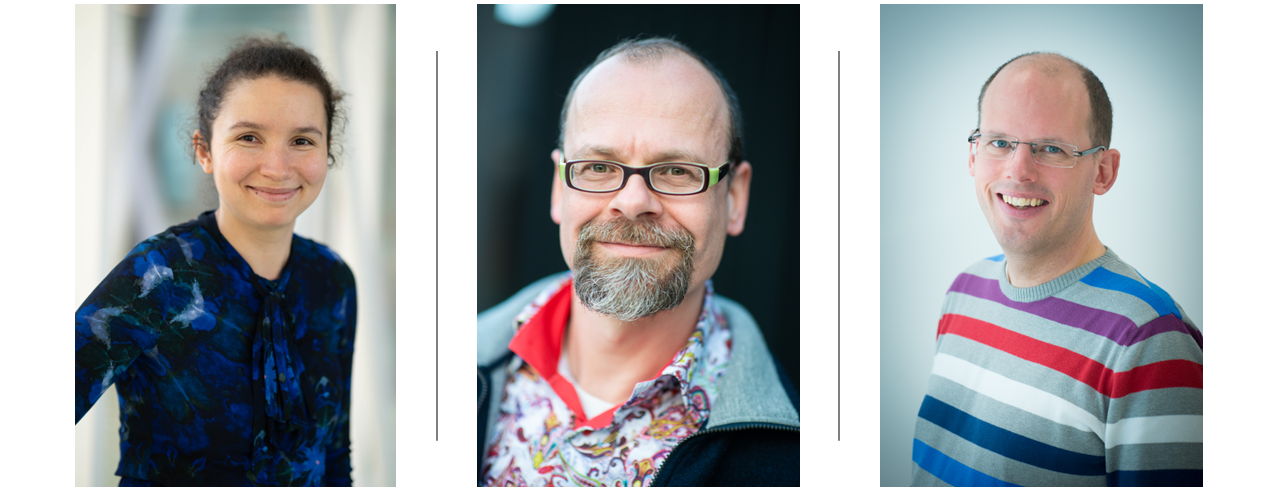
Enter AI
There is one big issue with this proposal: time. It seems unlikely that many researchers will have the time to painstakingly proofread their metadata entries.
"This brings us to the final piece of the puzzle," says Alexander Botzki, Lead of VIB Technology Training and organizing committee member of the fifth Applied Bioinformatics in Life Sciences conference, who contributed to the research, "and that's AI. More specifically, large language models like the one used by ChatGPT. While these models have their issues, they are very good at taking over tedious proofreading tasks and creating structured outputs based on various sources of information."
As a proof of concept, the team developed a workflow in which GPT-4 read lab notebook entries, metadata files, and publications. The machine learning tool checked for consistency across entries using five keywords related to title, authors, topic, methodology, and repository. Based on this, a structured report can be generated that details the degree of similarity between entries, which is then used to identify and correct likely errors.
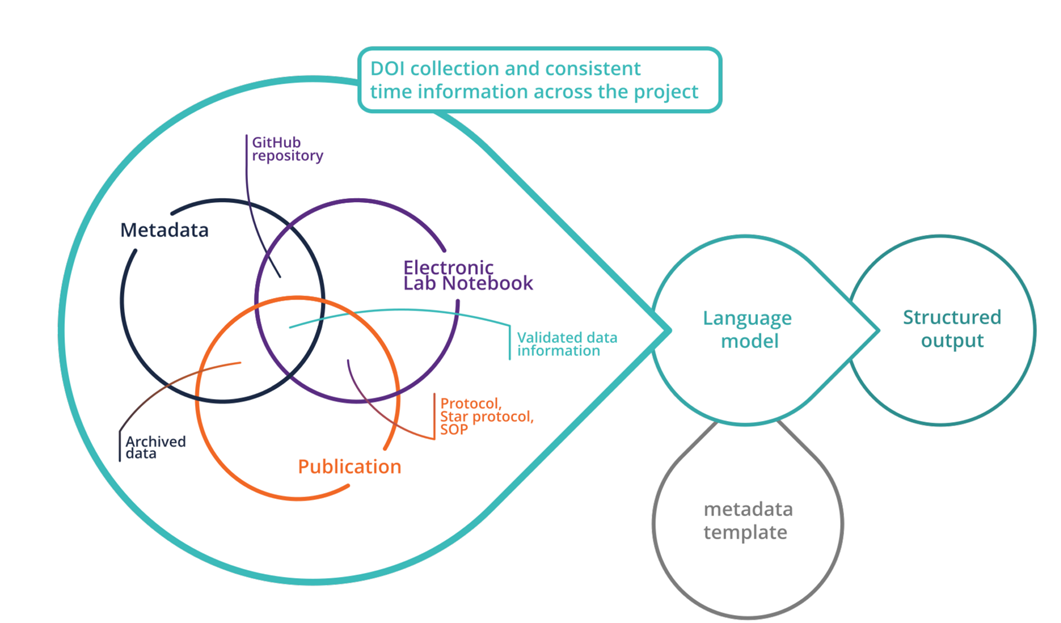.png)
One major additional advantage of this method is that it tracks a project’s shifting goals over time. Through keeping tabs on all stages of a research project – lab notebooks in the beginning, metadata in the middle, and manuscript at the end – the AI-mediated workflow can more easily correct and complete the documentation through different layers of openly accessible and inaccessible records.
"In essence, this approach is beautiful in its simplicity," says Woller. "Combining the redundancy with AI-powered proofreading allows researchers to reduce reporting errors, improve reproducibility, and, ultimately, champion the FAIR principles in bioimaging."
Looking to dive deeper into the world of applied bioinformatics in life sciences? Join us for our upcoming conference on the topic.
Publication
Woller et al. What we can learn from deep space communication for reproducible bioimaging and data analysis. Molecular Systems Biology, 2023.
Want to be kept up-to-date on our biotechnological news and stories? Join our community and subscribe to our bi-monthly newsletter.
Gunnar De Winter